At a glance
- Deploying AI technology in a workplace must be done sensitively for it to be recognised as a positive, and not a threat.
- Used effectively, it frees up time for employees to take on more value adding work.
- Applications of AI in medical research have grown in number and complexity.
When the National Health and Medical Research Council (NHMRC) first began looking into developing machine learning algorithms that would look after a time-consuming and laborious task, one of the greatest concerns was how it would make staff feel about job security. Management in the Australian Government agency knew the technology would have to be deployed sensitively for its arrival in the organisation to be recognised as a positive, rather than a threat.
When the project moved beyond proof of concept and the technology began to be scaled out into the real world, such worries diminished.
“Once it was released, we couldn’t stop the staff from using it,” says Tony Krizan FCPA, NHMRC CFO and CIO.
“They came to realise that it saved them a bucket-load of tedious work, and it meant they could get on and do the sort of work they have always wanted to do.”
What is that “tedious work”, exactly? Actually, it is work that is at the very core of the NHMRC and, as such, it is essential to get it right.
The NHMRC, among other functions, funds health and medical research in Australia. Researchers apply for funding for projects, and their applications must be considered by independent and expert individuals or panels of specialists. If the research focuses on a particular type of cancer, for example, the application must also be considered by an independent expert with great knowledge in that area.
The number of applications has grown dramatically and, due to the nature of medical knowledge, their complexity is also increasing. It was recognised that technology that would help match an application with an expert would be valuable.
“We had to fairly aggressively search out how to leverage available technology,” Krizan says. “That led us to explore how to automate the sorting and assigning of applications to peer reviewers and peer review panels.
“We receive thousands of applications every year. For each one, we need to find the most appropriate reviewer, someone with the right scientific skill set. There are many more thousands of reviewers than there are applications, and we not only have independent expert reviewers, but we also have review panels.”
What's behind the machine learning technology
The system developed to match applications to reviewers is based on semantic analytics technology, which has already had an astounding effect on the process for which it was built.
The wholly human process took 20 people more than 500 hours to complete. The new system can do the same job in six hours – the system takes a few seconds; the rest of the time is taken up by one human conducting quality assurance.
Krizan defines the underlying technology as “low-level machine learning”. Machine learning is a subset of artificial intelligence (AI) and was deployed using active, supervised teaching of a computer system to search for and recognise particular data points to develop recommendations.
On a basic level, the system searches the text within research funding applications, figures out what the research is about, and matches that application to an expert or experts in that exact subject area. A human quality controller then checks the match.
In this case, as mentioned, the machine learning involves semantic analytics.
A good definition of this, Krizan says, comes from the Expert System website: “…the process of understanding natural language – the way humans communicate – based on meaning and context ... It analyses context in the surrounding text and analyses the text structure to accurately disambiguate the proper meaning of words that have more than one definition.”
“That is quite a wordy definition, but it’s a good one because it’s quite articulate,” Krizan says. “In short, the machine is able to read words and it’s able to work out what those words mean in their intended context.”
Understanding the value proposition before building the technology
From an organisational management point of view, Krizan says, it is incumbent upon leadership to actively explore how technology can be leveraged. If businesses can learn from each other, that learning cycle can be shortened.
Accordingly, the Australian Government has recognised and begun promoting the NHMRC’S work. The NHMRC itself has committed to making the technology, and the knowledge of staff such as Krizan, available to other departments.
“The thing about technology is you’ve really got to embrace the notion of ‘build it once, use it many times’,” he says. “It’s just a matter of designing a system to enable it to be flexible enough to be leveraged in other places.”
What about privacy issues? Is combining machine learning with historical and current records a recipe for disaster?
“One of the biggest concerns I have is around human rights and privacy, so we’re putting a very strong control container around this technology,” Krizan says.
“We’re doing a privacy impact assessment and we also have to think about intellectual property.”
If there is a single most powerful lesson he has learned throughout this process of introducing new technology, Krizan says, it’s that it is not just about the task of understanding the value proposition and building the technology. It’s also about managing and retraining staff, implementing a change program, managing social expectations and identifying issues that could arise when disparate personal data is brought together by powerful technology.
While the offering that comes with machine learning is a potent one for an organisation, the risk is also considerable. “Consider just the staff angle,” he says. “They’re busy people who already have a day job and you’re saying to them, ‘We’ve got a new tool for you. You need to change your standard operating procedures and when you do, life will get better’.”
That process of change takes energy because it involves an organisational shift, not just a new system.
“Your business will look very different 12 months after you launch the technology because it comes bundled, by necessity, with an organisational transformation.”
Gaining AI knowledge: How Tony Krizan FCPA became an expert
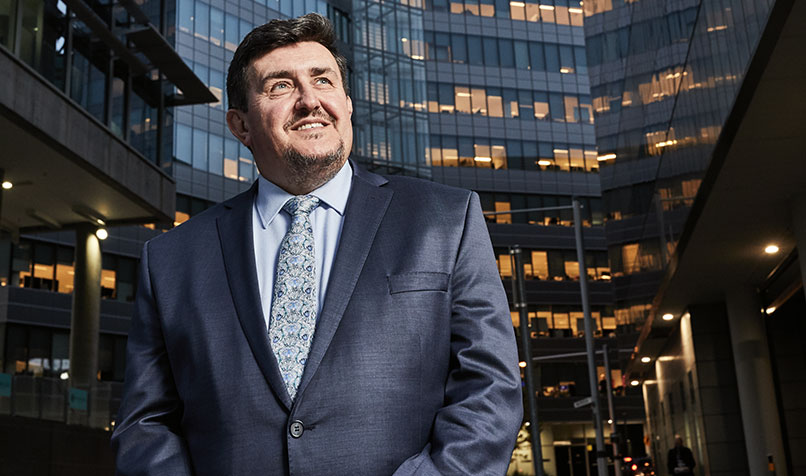
Tony Krizan, until recently a stranger to AI, in less than 12 months became enough of an expert to recognise the opportunity offered by the technology and to use it to set a new strategic direction for NHMRC. Here’s how.
“Several years ago, I attended a conference and was in a room full of very smart CIOs. I came back and said to my boss that even though we’d always had a lot of data and sophisticated reporting, we didn’t really do data science.
“I said, ‘Right, we’re going to do this’, and my suggestion was met with some amusement, but I persisted. It took me about 12 months to develop a good enough understanding to get started.
"The thing about technology is you’ve really got to embrace the notion of ‘build it once, use it many times’.”
“How did I do that? There is a vast amount of incredible information online. I spent the first hour of every morning reading blog feeds from leading data scientists and other information sources. I absorbed information from wherever I could find it. Leading data scientists are incredibly sharing, so there’s an enormous amount of free information online.
That’s really how I did it! If I could point you to one website, it would be datasciencecentral.com as one example of an incredibly useful information source. It will open up an entire world to you. If you invest time to understand the technology and how to deploy it, there is great potential to realise efficiencies and increase effectiveness.
Ultimately though, it would be remiss of me not to recognise that the critical factor to our success was finding a specialist technology partner that shares our vision and is committed to working closely with us during this transformation, and for which I am grateful.”